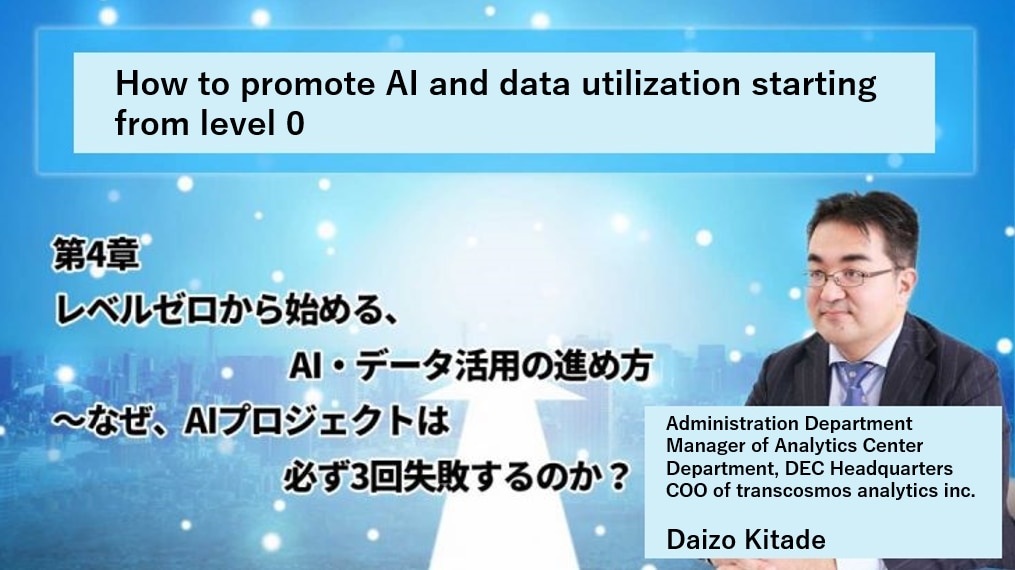
The Cutting Edge of Contact Centers - Chapter 4 -
Table of contents
|
Call center is the forefront of digital transformation.
In recent years, we have heard more and more words like digital transformation and the 4th industrial revolution. Digital transformation is the concept that "Spread of IT changes people's lives in all aspects for the better" proposed in 2004 by Professor Erik Stolterman of Umea University in Sweden.
According to the Survey on the Current State of ICT in Our Country
(link available in Japanese only)by the Ministry of Internal Affairs and Communications, digital transformation refers to companies "use digital technologies to create new products, services, and business models, and create value and establish competitive advantages by transforming the online and real customer experience."
At any rate, it refers to the movement to create new industries and customer experiences with the latest digital technologies, and they say that call centers and contact centers will be greatly affected by digital transformation.
However as shown in Figure 1, you could say the whole history of the call center and contact center has always been exposed to and evolved under the influence of digital transformation from its inception to the present.
Theoretically speaking, the idea of CS-oriented management typified by the aforementioned "Goodman's theories" have spread, and technically, the launch of the NTT Call Forwarding Service led to the emergence of the so-called customer service center. With the IT revolution and the Financial Big Bang, it grew rapidly and came to be called as call centers. Moreover, with the spread of the Internet, smartphones, and social media, it expanded as contact centers that can respond other channels in addition to telephones. Now we are being asked to take measures to use big data and AI.
Long before the term "digital transformation" was coined, call centers have always faced and been at the forefront of the latest digital technologies.
Figure 1: Call center evolution history is the digital transformation history.
However, these days I often hear some media saying, "With the advent of AI, call centers might disappear in 10 years." The discussion was sparked by the research by Professor Osborne of the University of Oxford that listed call center's telemarketing as one of the typical "Jobs That Will Disappear in 10 Years by AI."
Personally, if I was asked whether call centers would really disappear in 10 years, I would say "No!" promptly, but It's not uncommon to see people arguing that "Is AI your enemy or your ally?" with taking secondhand information seriously. Also those who like such discussions seem to lack basic knowledge and practical experience about AI.
Fortunately, the recent AI boom has faded in around 2019, and I feel that it has passed the peak of excessive expectations like the bubble tea fad in recent years. We are now entering the stage of thinking about how to respond to customer needs and how to use them to resolve problems at the site from the practical point of view. In fact, until a few years ago, we received many inquiries that "What can you do with AI? (If using AI to produce results, anything is fine.)" These days, there got more serious companies which consult us about practical use such as, "Which AI can be used to resolve these problems? (It does not have to be AI if we get results,)" which seems more sound thinking to me.
I think people who still appreciate the word AI should have a second thought about it. Today's businessperson should not be free enough to be swayed by fake news.
How to proceed with AI and data utilization project from level 0
Still, the contact centers that play a leading role in digital transformation must confront the challenge of utilizing AI and data.
According to a survey conducted by Nikkei Computer and Nihon Keizai Shimbun on the state of AI use in 113 major companies in Japan, in 83% of the companies AI utilization was a matter of course, and 60% said AI utilization exceeded their expected performances. However, only about 10% of the companies had enough data they need, and 88% considered it as a challenge to augment their human resources of AI utilization. Even though Japanese companies recognize the need for AI, they do not have the data necessary for AI, and there is a shortage of human resources that can tackle and promote this situation.
For companies that do not know where to start and how to move forward with use of AI and data, transcosmos has prepared the "level judgment table for AI and data utilization" as shown in Figure 2.
In general, AI and data utilization projects start with a small special team, conduct limited trials, and create a local successful experience. Then we share the case with other departments, and create a state that the site staff can operate by themselves at multiple sites. Ultimately, we restructure the entire business upon the assumption of using AI. In order to start an AI and data utilization project from level 0, it is necessary to acquire basic AI knowledge and define specific utilization tasks.
In this note, I would like to briefly explain the basic knowledge and the key points for defining tasks.
Figure 2: Level judgment table for AI and data utilization from level 0
When I was asked to give a lecture on AI, firstly I started with a story that there are 2 kinds of "AI."
One is "general AI," which has the ability to understand and judge autonomously like humans. It's like the certain nationally popular robot cat. The second is "specialized AI," which supports some of the specific human tasks. Smart speaker and chatbot are categorized into this group.
Important thing is to note that "general AI" is currently imaginary, and vast majority of AI in practice is "specialized AI" only. People with excessive expectations about AI often do not understand the difference between the two AIs.
"Specialized AI" is not new and has been around for quite some time. I like role-playing games, and the first time I encountered "specialized AI" was in junior high school. It was a combat AI which autonomously controlled friendly characters and fought for me. However, AI of the time did not have learning ability, and had some fatal problem such as, the monk on my side continued to chant an instant death spell that did not work against the Great Devil until all of us were wiped out.
Now the latest AI has acquired that learning ability. By accumulating big data and processing it at high speed, the specialized AI appeared, which can learn how to recognize a picture of cat from a large number of images, convert telephone conversations into text, predict customers with high purchase possibility, and control conversations and actions like chatbot and automatic driving.
All of these specialized AIs are based on "supervised machine learning."
"Supervised machine learning" is a technology that learns repeatedly from training data in the past, discovers hidden patterns, and applies these results to unknown data to give predictive scores. In order to develop the specialized AI, we should define tasks that we want AI to resolve with taking into account the characteristics of the company and industry, and to prepare the necessary training data to learn. On the other hand, the algorithm itself used for prediction have already been released as a tool or available for free on the Internet.
In other words, what is important is not the AI algorithms, but the training data which adapt to the defined tasks to make AI learn. That is why there is a saying "Data Is New Oil" (just as oil was the source of economic wealth in the 20th century, the oil in the 21st century is data.) Based on the survey result that "only about 10% of the companies have enough data they need" mentioned above, we can see how many Japanese companies do not understand the importance of training data which adapt to the tasks to be resolved by AI.
Reasons why we fail to "define tasks" for AI and data utilization
I kept saying that training data adapted to define tasks to use AI and data is important. Nevertheless, I must say actually the "task defining" is a troublesome work, which is "easy to say but difficult to do."
For instance, if someone asks you a question that, "Let's define specific direct marketing and call center tasks that you want to resolve using AI and data in your own company!" What tasks do you imagine?
Here are some common examples.
1. Cancellation prevention: You want to anticipate customers who are likely to cancel.
2. Stock prediction: You want to predict stock for inventory control.
3. Prevention of job separation: You want to detect and stop workers from leaving the jobs.
4. Reminder of unpaid due: You want to predict defaulters.
5. Telesales for corporations: You want to predict companies that are highly likely to contract.
6. Sending catalogs and DMs: You want to send only to those who are highly likely to purchase.
7. Call log summary: You want to automatically extract and summarize VOCs from the conversation text of speech recognition.
8. Cross-selling/Up-selling: You want to visualize customer feelings of purchase intent with emotion recognition.
And there are many others. However, they were all very common examples that "failed" to define tasks in AI and data utilization. "Task defining" is not that easy.
As an example of call center's effort to prevent cancellations, simply predicting the possibility of cancellation is not enough to start a last-minute sales pitch when they take an inbound call for canceling from a customer. A person with practical experience in cancellation prevention will find it necessary to understand the customer's reason for cancellation and to detect the similar persons who want to cancel because of the same reason for an effective sales presentation.
Besides, if they give outbound calls to customers to prevent cancellation, early customer care and retention measures for customers who indicated signs of cancellations will rather increase the risk to cancel. It is what's known in the business as "to wake a sleeping dog." It is a phenomenon that such a call gives them an opportunity to cancel. In order to reduce the risk, we need to predict the "possibility of waking up the dogs" and to remove this segment from the calling list so that they will not get calls.
Likewise, in AI project they often find that what they want to predict is not a vague probability of cancellation, but another indicator. Then they have to reconsider the objectives or subjects of prediction and prepare a new system since just predicting will not generate measures. In many cases, if the prediction accuracy is low in the practical prediction, they will restart from a reconsideration of other utilization objectives and re-collection of training data.
These "task defining" failures can be summed up in 3 reasons, as shown in Figure 3. There are quite a few cases that we want to use AI but just can't face the tasks head-on because of "lack of knowledge" or "technology first" mind. "I want to realize the next-generation communication using AI and data!" If you start a project with that mindset, it always fails. The reason is simply that the idea of "doing something with AI and data" triggers the failure of "technology first" in the first place.
Figure 3: The reasons why we fail to define the tasks of AI utilization
However, no matter how right the knowledge and attitude are, they still may fail in AI and data utilization projects. It is because they continue the project without knowing that the first defined task is not a "real task" in most cases and the project comes to end without noticing it. The failures happen commonly because they define tasks without a view to the actions at the site.
Accordingly, we need to learn the right thinking process to get to the "real task" and how to proceed with AI projects. As shown in Figure 4, transcosmos recommends the way to proceed project with separated 3 trials of PoT, PoC, and PoV.
Figure 4: How to proceed with AI projects to reach "real tasks"
PoT (Proof of Technology) is a free demo or trial done in such case as, "We have never seen or used new technologies like AI, so without purpose we want to try and compare various technologies as much as possible!"
PoC (Proof of Concept) is a trial done in such case as, "We want to judge the feasibility of our concept or idea after defining the tasks we want to resolve and introducing the prototype technology on trial!" This trial is often for a fee.
PoV (Proof of Value) is a paid trial done in such case as, "We want to compare the pros and cons and system differences between existing and newly developed products, technologies, and systems, and want to evaluate ROI by introducing into part of work for a short period on trial before fully introducing them!"
A fairly common mistake is to repeat PoT with the intention of "PoC." This tends to happen in AI projects in the R&D department. I also see another case that, under the instruction of the management which drives use of AI enthusiastically, the site introduces AI on an ad hoc basis and carries out PoC or PoV without setting definition and criterion of the success beforehand. These failures occur because they don't understand the differences between PoT, PoC, and PoV and misuse them.
If the content of technology is unclear and we cannot imagine how to apply, we should perform PoT first and supplement the insufficient knowledge during the process. Also since we often reach the "real task" after practical trials, when we doubt if we could have found a "real task," we should start PoC small with an emphasis on speed and then move to PoV at the time we are certain that the "real task" gets clarified.
In this way, it is the rational way for proceeding with AI project to choose PoT, PoC, and PoV for different purposes, and control the risk by experiencing "3 times of failures" in each phase and review the plan from learning.
The problem is whether the AI project personnel's perseverance and enthusiasm lasts to the end, and whether the management which is skeptical for using AI or fast-and-sloppy and the operational sites which involved in frequent trials accept to "repeat failures at least 3 times" and wait for good results. There must be a mountain of AI projects that were closed due to lacking people who are willing to use AI and the corporate culture that accepts such challenges. Honestly I feel sorry for these projects.
Practical examples of AI projects in the next-generation contact center
I will show you an example of AI project produced by transcosmos that optimizes the LINE message distribution by segment in online shopping business.
The general online shopping company used to distribute messages to all their friends on LINE for stimulating purchase, but they had a problem that there were too many fruitless messages and the cost performance was bad.
So, transcosmos proposed the concept of the message distribution by individual segment with "supervised machine learning," which enables them to distribute not indifferently but individually to "likely buyers" at "the time they may read it" as much as possible. Specifically, in order to enable LINE message distribution by segment targeting at "likely buyers" only, we used "DataRobot," a specialized AI which automates machine learning, to build a statistical predictive model that automatically learns "buyer" patterns from purchase history and browsing data, and give purchase possibility score to each individual.
Using DataRobot was good that we could easily predict the future, but that was insufficient as a system to implement at the site. This was because, as shown in Figure 5, it is a hard task to every time manually input data which is accumulated and updated, reconstruct a predictive model, and distribute messages. To continue to operate instead of ending in a temporary project, we needed to automate not only the prediction but also the entire distribution work system.
Figure 5: We need not only prediction, but also the automatic distribution system.
So we introduced "DECode” (link available in Japanese only)which is a unique DMP (data management platform) of transcosmos to automate the integrating and processing of multiple databases. The generation and tuning of forecasting model of the target segment are automated by Datarobot. In addition, we developed our original business tool "WHITE BASE" (link available in Japanese only) that automatically distributes LINE messages to the selected list of the highly likely buyers.
The overview of the efforts above is shown in Figure 6. This system was very effective and the ROI was 4.3 times of conventional full distribution.
Figure 6: Example of list creation for LINE distribution by segment in online shopping business
However, that was not all of this project.
As we continued to operate this system, we faced a new challenge: simply targeting the highly likely buyers and harvesting them like "slash-and-burn farming" would eventually exhaust the customer list. To avoid this, we introduced an additional measure that harvests short-term sales from the highly likely buyers with additional message distributions, and uses coupons to cultivate the unlikely buyers into the potential highly likely buyers.
At the same time, another problem occurred that even after we send LINE messages, the opening and browsing rate did not increase. Therefore, we developed a model that predicts not only purchase possibility but also "optimal distribution timing" and incorporated it into the existing system. As a result, within two days after the distribution, the website inflow increased by 1.2 times and the purchase rate increased by 3.4 times.
It is not rare for AI projects to have twists and turns like this. Almost always, just introducing AI is not enough. Some additional work definitely arises including reviewing and adding tasks, making up for tasks manually, developing and operationalizing peripheral systems like BI and automation tools. To create an acceptive corporate culture for using AI, I think not only promoters but also management and operation site staff should know that is the way of AI project.
Welcome to the next-generation contact center.
What I wanted to say in this chapter is summarized in the following 3 points.
1. To prepare for digital transformation, we should correctly understand the latest technologies like supervised machine learning, and accumulate training data necessary for AI to learn.
2. "Task defining" is still human's work. We need to promote AI projects that correctly use PoT, PoC, and PoV and identify the "real tasks."
3. Introduction of AI alone is not enough, and mostly we are required to review human roles, business processes, work styles, and to develop BI and automation tools.
Well, if we ask someone who has ever worked on an AI project at the contact center whether「 would really eliminate call centers in 10 years, then what would they say?
The important thing is to face the reality that whatever we use speech recognition, chatbot, or machine learning tool like DataRobot, some work can be done by the current AI technology, while other work cannot (the work that should be done by humans.) I think the idea of "AI takes the place of human" is unrealistic, and as shown in Figure 7, the future will be a form of collaboration in which people think of tasks, have AI learn them, and AI takes over part of work.
Of course, some may disagree with my idea. I hope you actually participate in AI projects and get to your own answers.
Figure 7: Some work can be done by specialized AI, while other work cannot (the work that should be done by humans).
Meanwhile, it is important to inform you that all business companies do not have to carry out these AI projects on their own from the start.
I don't mean to advertise, but I think it is a good option for a business company to use an outsourcing service that integrates AI and operations like the services of transcosmos. It's a very smart strategy to develop core AI on your own and outsource the rest.
Just as described, I would like to suggest that the future management and AI promoters will need a strategic thinking to outsource after prioritizing the scope of AI utilization.
Postscript
Modern companies, and also contact centers have to implement digital transformation because they need to prepare for the diversifying work styles due to entering the age of "super" labor shortage, and highly developed technologies due to the development of AI. How will the world of the next-generation contact center be beyond the innovation?
Some people imagine a world managed and dominated by AI, a dystopia, and grieve for the future. However, I am somewhat optimistic. I think AI does not take jobs away from humans. On the contrary it gives human a chance to take back the "humanity."
By making AI learn, letting AI help people, and cooperating with AI, humans can concentrate on the work which can only be and should be done by humans, and take back the human nature. How about regarding AI with less hostility and trying to accept it as a cooperator working together?
We can only do that by digitally transforming the work style, the thinking, and the attitude of ourselves. When we finish the mind revolutions, maybe it means that we are standing in front of the entrance of the "world of the next-generation contact center."
transcosmos are always very happy to be the best digital transformation partner for everyone who are heading for that "world."
transcosmos is a global and one-stop solution provider that offers digital marketing, 5A analysis, eCommerce and contact center support that adds value through optimizing cost and driving sales. For more information, please feel free to email us at; |
Let’s talk ✉:sales_global@ml01.trans-cosmos.co.jp |